Call of Duty: Modern Warfare II is a AAA game on PlayStation 4 & 5, Windows, Xbox One, and Xbox Series X/S. DI4D worked closely with Infinity Ward (Activision) to deliver the facial animation for the best-selling video game, DI4D had previously delivered twenty-five minutes of facial animation for the cinematics in Call of Duty: Modern Warfare (2019). This time, DI4D used its new PURE4D solution to process over four hours of facial performance data for both cinematics and in-game animation, making it DI4D’s largest-scale project to date.
4D capture for improved digital double facial rig creation.
In order to obtain the highest possible level of graphical realism, Infinity Ward committed to an animation pipeline in which the in-game characters are digital doubles of the actors who play them. Such as Colonel Alejandro Vargas who is played by Alain Mesa. Vargas is a Tier One Mexican Special Forces Operator who is the leader of Los Vaqueros and an allied member of the elite counter-terrorism unit Task Force 141.
The image above shows the test shoot for the actual performance recording. The team did not require the actor to have dots on their face.
The actors were captured with simultaneous performance and audio on the stage, the actor’s body, facial and vocal performances were all recorded simultaneously. The team used eight Technoprops HMC units over Wi-Fi on the stage. The team could record fix or download data via a dedicated 10-gig switch. For example, on the last performance capture shoot day, the team captured over 1 TB of HMC videos.
In terms of the rig and pipeline this HMC footage fed into, this first required the 3D shape and appearance of all fourteen of the main cast to be captured in super high detail using Activision’s Light Stage system. A Light Stage scan of each actor with a neutral facial expression was retopologized and used by Infinity Ward to infer automatically an initial FACS-based animation rig for that actor using its FaceGEN system. “We usually just do one session in the light stage (per actor),” explains Bernardo Antoniazzi, Principal Technical Artist at Infinity Ward. “We capture up to 100 poses, the standard basically FACS, poses, and then on the same day, we also do a DI4D capture. We go from the neutral to the pose – so we have the full range of motion – of every kind of deformation. We rely on the 4D data to build the rig”. The DI-4D uses nine x 12 megapixel cameras.
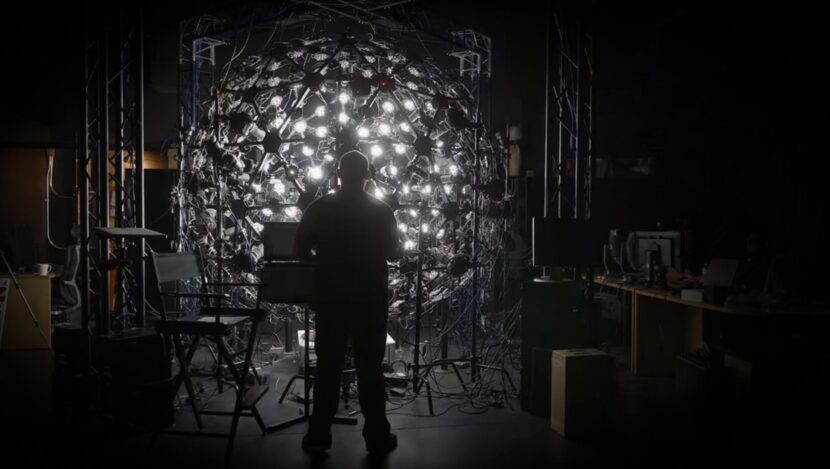
Next, DI4D captured an extensive series of dynamic facial expressions and several phonetic pangrams of each of the main cast with a DI4D PRO system. Phonetic pangrams are phrases designed to include all the 40 basic sounds or phonemes in the English language. The DI4D PRO system comprises nine synchronized, 12-megapixel machine vision cameras, running at 30 frames per second. As such it is able to capture a large number of subtle ‘in-between’ dynamic facial expressions.
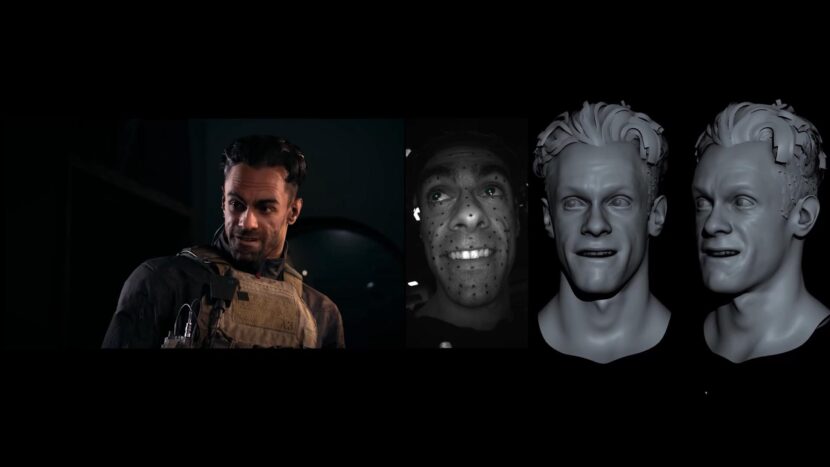
DI4D processed the machine vision camera data first using photogrammetry to produce a raw 3D scan sequence, in which a separate 3D scan is reconstructed for every frame of the video data. The retopologized neutral expression mesh from the Light Stage was then registered precisely to one frame of the raw 3D scan sequence data in which the actor has a similar expression to the light stage neutral. The registered mesh was then tracked accurately through the whole 3D scan sequence using optical flow to apply a consistent mesh topology to every frame in 3D scan sequence. This results in 4D data that is represented as dense point cache animation.
One of the main advantages of DI4D PRO data is that it captures a large number of subtle co-articulated facial shapes, not just a sparse set of extreme expressions associated with traditional FACS scans. Infinity Ward used the DI4D PRO data to supplement and refine the initial FACS-based rig they created for each actor. This approach to facial rig creation resulted in a huge time saving compared to a traditional rigging process involving sculpting 100s of facial shapes. It also ensured that the facial rig was able to more accurately represent the full range of the actor’s facial expressions, allowing artists to focus on finer details that would not normally receive any attention.
Above shows (in the centre), the machine vision data processed as a Raw photogrammetry solution and then re-topologized to a neural mesh from the Light stage data (R). On the left is some of that light stage pose data. One of the main advantages of the 4D approach is that you obtain subtle, coarticulated shapes and not just a sparse set of extreme facial expressions, which are normally what is output with a traditional isolated set of 3D FACS scans or poses. The mesh is effectively tracked through the whole sequence using optical flow, so you get a corresponding consistent topology mesh.
Driving digital doubles with high fidelity, in-game facial animation
The facial performances that were used in the game were captured at Infinity Ward’s dedicated Performance Capture (PCAP) stage. For every scene, facial performance data was captured using Head Mounted Camera (HMC) systems simultaneously with the audio voice performance and body motion capture. The simultaneous capture of all of the elements of performance from the same actor was important to achieve accurate synchronization and the high degree of realism the team that were after.
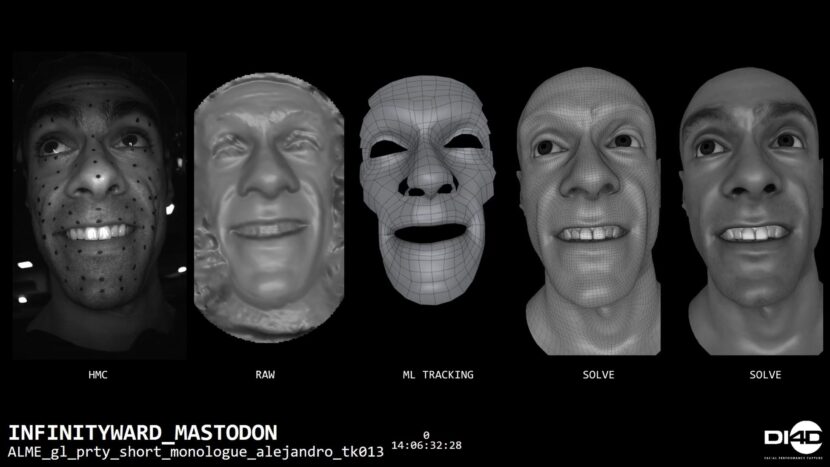
One of the key advantages of this pipeline is how objective the team makes the process. The final animation is evaluated objectively against the actor’s actual facial performance. This contrast with a pipeline where the actor’s performance is re-target to a different character, which becomes a much more subjective process based on the animators and visual effect supervises opinion. This pipeline puts much greater emphasis on capturing the straight performance as opposed to then adjusting it in post, which is especially important given the volume of the work the team were trying to do. The actors also respond well to this workflow, knowing that their performance will be faithfully reproduced in the final game.
DI4D processed hundreds of selects made from the HMC footage using its new proprietary PURE4D solution. PURE4D combined the actors’ facial performance data captured with HMCs during PCAP with the higher fidelity dynamic expression data captured with the DI4D PRO system. PURE4D also uses advanced machine learning algorithms to reduce significantly the amount of manual clean-up normally associated with processing 4D data, hence allowing 1,000s of seconds of high-quality facial animation data to be delivered in a timely manner.
The advantage of this speaks for itself, what took three weeks prior to model, now takes one day, and even after that three weeks, there was often still the need for a validation process. In terms of the facial animation what had previously taken two weeks can now be done in three days. As mentioned, this method also avoids the inconsistencies from the subjective interpretation of key aspects of the prior way that the team did things. The team found that the new method removed this subjective interpretation step, and allowed the artist to focus on making meaningful improvements rather than just fixing problems. It reduced the amount of clean-up and increase the accuracy of the final defamations.
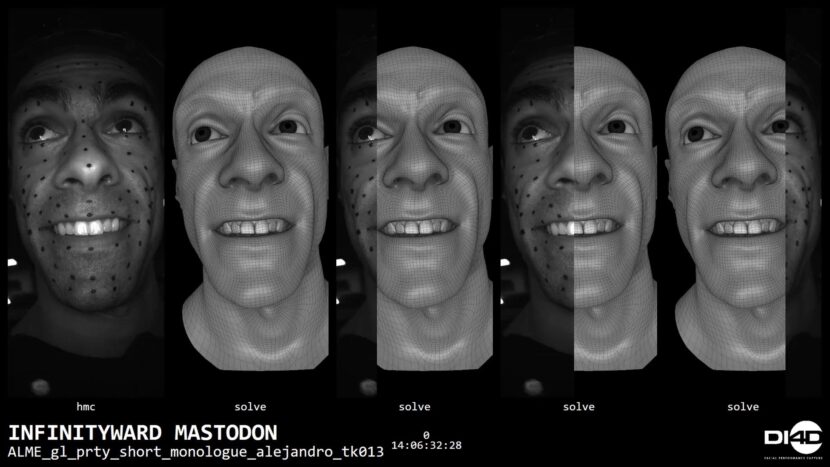
The machine learning (ML) was particularly of interest. The team used of series of blend shapes encoded into a lower dimensional space representing the bones and linear blend skinning. This method of blend shape compression allows for efficient performance while also maintaining high fidelity in the game engine. Blend shapes are expensive to run and do not scale with respect to multi-platform deployment (- with the appropriate level of fidelity), especially on low-end platforms when you have facial rigs that have over 300+ high-resolution blend shapes.
“DI4D technology allowed us to implement incredibly realistic facial animations in a way that saved us a lot of time in the production process,” commented Sean Comer, Principal Machine Learning Engineer, Infinity Ward. “This meant we could focus on other important aspects of the game’s development without sacrificing the level of immersion and detail we were aiming for.”
The type of point-cache animation generated by PURE4D is able to faithfully represent an actor’s facial performance with a very high degree of accuracy, but it can be very heavy for in-game use and difficult to edit manually. Infinity Ward addressed this by solving the point-cache animation to their rig’s animation parameters. This process introduced semantic control allowing an animator to modify the performance, giving final creative control to the animation team. However, because the rig and PURE4D data were both derived from the same set of DI4D PRO data, it was still possible to obtain a rig solve that was objectively very close to the point-cache animation and hence to the actor’s original performance. The rig-solve process also allowed the memory footprint of the animation to be scaled down for in-game use on a variety of hardware platforms.
Overall, “we create this sort of a loop where we go from the initial rig to the performance,” explains Antoniazzi. “We check if the rig can reproduce the performance with fidelity. If not, we take shapes directly from the performance and we ingest them back into the rig, – it is an iteration process.” This makes sure that the rig can produce everything correctly and with the required fidelity. “Basically, we make sure we can produce final quality animation in a ‘parametric’ way, with what we get from the performance (HMC) and with the rig built with the 4D data”.